SpatialDETR - Robust Scalable Transformer-Based 3D Object Detection from Multi-View Camera Images with Global Cross-Sensor Attention
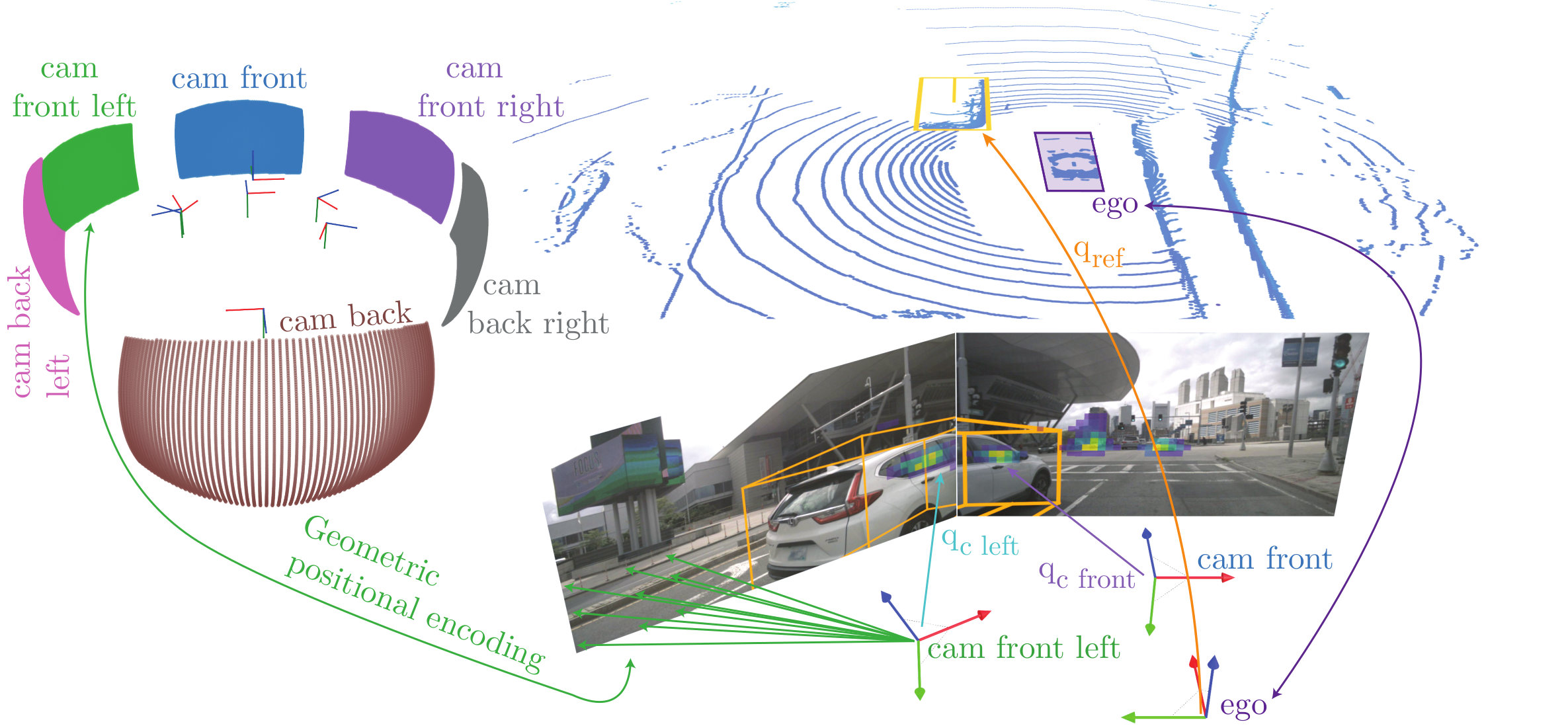
TL;DR: SpatialDETR is a method for robust multi-view 3D object detection that explicitly incorporates the extrinsic calibration by utilizing a novel geometric positional encoding. This allows for global receptive fields and attention across sensor borders.
Abstract
Based on the key idea of DETR this paper introduces an object-centric 3D object detection framework that operates on a limited number of 3D object queries instead of dense bounding box proposals followed by non-maximum suppression. After image feature extraction a decoder-only transformer architecture is trained on a set-based loss. SpatialDETR infers the classification and bounding box estimates based on attention both spatially within each image and across the different views. To fuse the multi-view information in the attention block we introduce a novel geometric positional encoding that incorporates the view ray geometry to explicitly consider the extrinsic and intrinsic camera setup. This way, the spatially-aware cross-view attention exploits arbitrary receptive fields to integrate cross-sensor data and therefore global context. Extensive experiments on the nuScenes benchmark demonstrate the potential of global attention and result in state-of-the-art performance.
Summary Talk
Qualitative Examples
Acknowledgements
The research leading to these results is funded by the German Federal Ministry for Economic Affairs and Climate Action within the project “KI Delta Learning“ (Förderkennzeichen 19A19013A). The authors would like to thank the consortium for the successful cooperation.”